A company’s website has become the new “front door” of a business — especially over the past two years. With the growth of e-commerce, advertisers have seen a tremendous increase in website activity and are looking for new ways to turn these visits into actionable and predictive insights that can drive sales.
But like all real-life processes, online user journeys can be complicated, messy and unstructured. There are many potential gaps in the information available to marketers, so brands require more than a dashboard overview to make the most of the data they have.
Machine learning can help a brand determine the likelihood of a new site visitor to convert, or estimate the forward-looking lifetime value of existing customers.
This is where machine learning can help. Machine learning models can dig deeper into the consumer journey to demonstrate things like what visitors do on a brand’s site, where they drop off, and what marketing campaigns resulted in purchases.
Machine learning models can also offer brands some great predictive insights. By learning from the actions of millions of previous visitors and customers, machine learning can help a brand determine the likelihood of a new site visitor to convert, or estimate the forward-looking lifetime value (LTV) of existing customers.
Marketers still have a long way to go when it comes to harnessing the potential of machine learning. We often see hesitation to implement these models because they seem too complex, and the link to their business value is not always clear. Here, we break down the process of implementing machine learning models in three simple steps.
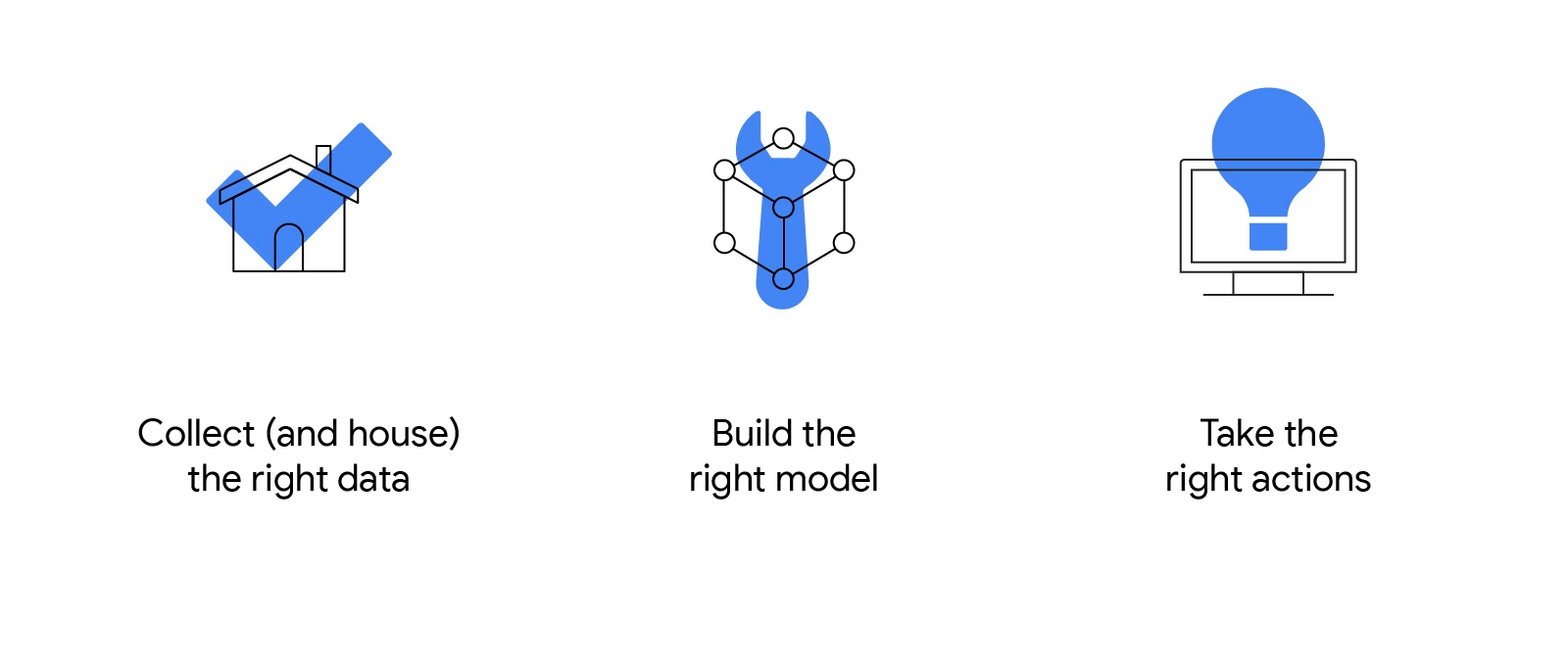
1. Collect (and house) the right data
With millions of diverse data points to work with, it's imperative that marketers start by narrowing down their business use case and collect the right data. This can be done by determining exactly what they wish to predict.
Do you want to determine which users are more likely to convert? Collect data on site events, user clicks, page visits, and whether or not they converted.
Do you want to determine what could reduce service subscription churn? Collect data on user-business interactions, their frequency, products owned, and whether or not they churned.
Google offers a wide range of platforms, tools and infrastructure to do that. Google Analytics tracks and records website hits, traffic sources, session data and conversions among many others. This data can be exported to Google BigQuery on a daily basis. BigQuery is a cloud data warehouse that lets you run super-fast queries of your datasets, and prepare them in a form that’s understandable and consumable for training machine learning models.
2. Build the right model
A machine learning model is a generalization that is learned from past data. Using dozens of signals from how users interact with your website (see previous step), one can develop models that predict likelihood of conversions for site visitors. These models learn from and work based on a wide range of signals, such as actions on site that determine site activities that can lead to a greater likelihood to convert.
Creating these models has many similarities to natural human learning activities; lessons, quizzes, and final exams. To build a model, the data from the previous step is typically divided into three sets:
- Training set (lessons), where the patterns and relationship between data elements are discovered.
- Validation set (quizzes), where the model is adjusted and tuned.
- Test set (final exam), where the model is tested against unseen data and its prediction performance is evaluated.
BigQuery ML bridges the gap between your website data and AI models by creating and executing machine learning models in the cloud. AutoML allows you to build custom machine learning models with an easy-to-use graphical interface.
3. Take the right actions
Once your team has analyzed the output of your machine learning model, it’s important to test and implement these learnings, so they can be used to improve your future business decisions.
For example, models that predict the likelihood of conversions can be applied immediately to your audience segments. They can help a brand to increase its return on ad spend by showing ads to the visitors they know are more likely to make a purchase in the near future.
Another example of activating these models is to use them to understand what elements and features of your e-commerce website provide greater signals and drive conversion actions. This can be used to inform UI and UX design decisions for your site. For example, if a visitor has recently added a product to their wishlist, or visited a certain product page, machine learning can help determine how much higher their odds of converting are.
Marketers are still in the early stages of implementing these models, and there are so many ways to use them. If brands can harness the tools available right now to analyze their data at scale, they’ll be able to gain actionable insights, filter out the unnecessary details and find new opportunities to reach consumers.