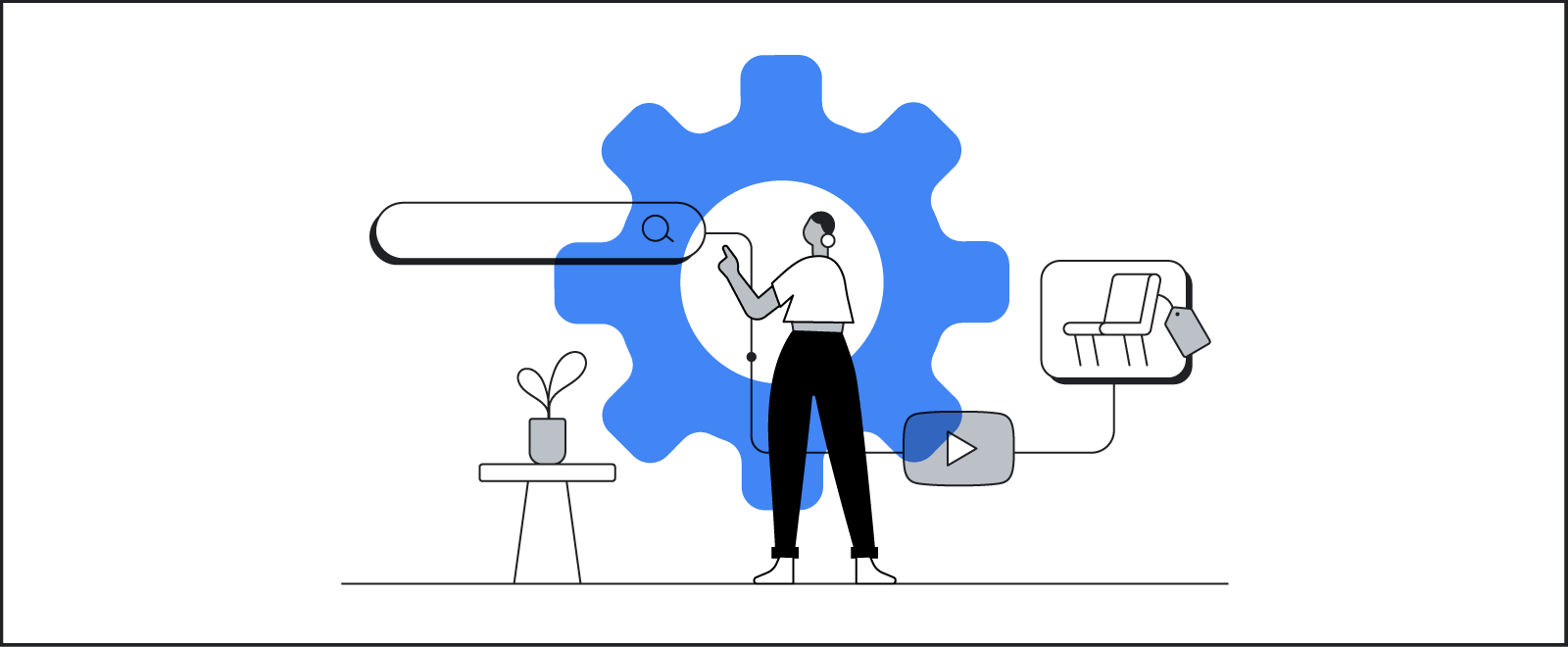
With the changing digital marketing landscape, data-driven decision-making has become more challenging ā and at the same time, more critical ā to preserve. As the industry evolves to adapt to a world that prioritises and upholds the privacy of users, the technologies used to measure and gather insights across the customer journey need to adapt alongside it. With observed data less available because of privacy regulations and restrictions, sophisticated machine learning models can help preserve measurement continuity while respecting user privacy and consent choices.
A secure and effective approach
Machine learning models are generated by analysing vast amounts of historical data, identifying correlations and trends between key data points, and using those insights to make accurate predictions about peopleās behaviour. Googleās models are rigorous. They are proactively validated and tested to ensure accuracy, have high thresholds for confident reporting, and are designed to be unique to your business. Most importantly, they put users and their privacy first, preserving their experience and protecting their data while you generate important insights.
With the changing digital marketing landscape, data-driven decision-making has become more challenging ā and at the same time, more critical ā to preserve.
Measurement-improving modeling capabilities exist in Google Ads to provide a more complete picture of the customer journey, including modeling for store visits, online conversions, and cross-device conversions. Last year, we announced predictive capabilities in Google Analytics 4 properties that use modeling to predict which users are most likely to purchase or churn based on historical data. Now, weāre bringing that same sophisticated and rigorous modeling technology to supplement your analytics data where gaps may be present, unlocking greater insight into the complete customer journey.
Closing gaps across the customer journey
First, modeling will restore behavioural data based on user and session metrics, such as daily active users and conversion rate, that may be unobservable when identifiers like cookies or user ID are not fully available. These data-driven predictions about peopleās behaviour will fill in the gaps, enabling uninterrupted measurement across platforms and devices, and more reliable, customer-centric insights in your Google Analytics 4 property reporting. Without modeling, you will have a less complete understanding of user behaviour on your site, with only glimpses into it based on the observed data you have available.
Second, conversion modeling uses machine learning to fill gaps in your conversion measurement that may occur because of discontinuity across devices or the absence of identifiers to unify customer journeys. The models assign linkages when your conversion events ā such as purchases, downloads, or sign-ups ā canāt be tied to a channel. Your conversion data will be modeled based on similar observed conversion paths to allocate conversions to the right Google and non-Google channels, such as paid search, email, or paid social in your Google Analytics 4 reporting. Modeled conversions allow for more accurate reporting, so youāre not left guessing where your conversions came from, and you can better optimise your campaigns.
Without modeling, you will have a less complete understanding of user behaviour on your site, with only glimpses into it based on the observed data you have available.
Once youāve determined where your conversions have come from, data-driven attribution models will help you understand the value of each marketing interaction preceding the conversion. Data-driven attribution uses data from your Google Analytics account to generate a tailored model for assigning conversion credit to paid and organic touchpoints throughout the entire customer journey. Using only the historical, consented, and first-party data available to you, modeling enables deeper attribution insights across the customer journey that can enhance your marketing ROI.
Modeling is central to privacy-safe measurement
As user privacy continues to be a top priority, and observed data becomes less available as a result, traditional methods of measurement have become less viable. You can, however, continue effective measurement while still prioritising user privacy by investing in first-party data collection, ensuring all your observed data is transparent and consented, and adopting solutions that use credible and validated modeling technology to fill in gaps when directly measured data isnāt available.
Get more value from your data and enhance your analytics by applying these modeling features and other privacy-safe measurement solutions coming soon to Google Analytics 4 properties.