Arn Knol is a commercial director at Dutch clothing company G-Star. He is responsible for transforming the brand's operating model with a data-driven approach.
A seamless retail experience across channels is important to both customers and brands. In the Nordics and Benelux regions, omnichannel shoppers make on average 2.9X more purchases, and spend 2.2X more compared to single channel shoppers.1
G-Star was founded in the Netherlands in 1989, so we’ve been around for nearly 35 years. Over that time, we’ve grown into a global direct-to-consumer retailer operating in 45 countries via a digital ecosystem, and a bricks and mortar and wholesale network.
As consumers expect more privacy-friendly online experiences, the need for first-party, high-quality, and accurate omnichannel data has grown.
Closing the data gaps would help us enhance our knowledge of what our customers want and how they behave across our entire digital estate.
But like many retailers, we realised we were facing data gaps between transactional and behavioural data. The shift away from third-party cookies and towards greater consumer privacy presents businesses with challenges in measuring effectiveness of marketing, especially across multiple retail channels.
At G-Star we see the opportunities this will allow by using first party data to help us create a competitive advantage.
To understand our customers needs and motivations we first needed to develop our data capabilities. Closing the data gaps would help us enhance our knowledge of what our customers want and how they behave across our entire digital estate. That's why we transformed our operational model, the way we work, and our attitude towards data.
Twinkle twinkle, data warehouse star
First, we wanted to ensure the right insights were available, real time, to anyone who needed them. In my view a data transformation is complete when everyone in the business has access to the data and can apply this data effectively in decision making.
Our first step towards this was to create a data warehouse on BigQuery. This allowed us to unify different customer data types and build a reporting layer on top. There was a vision that if we got all our data in one place, we would be able to create use cases — a way to identify, clarify, and organise system requirements related to a particular goal — on top of that data to create valuable insights from our data.
I’ll share some examples of use cases we created later on, but before we started to drive new or regular data sources into our marketing mix we needed to ensure we could trust our data implicitly.
For us, that meant looking at quality control. We asked the question — “Who's going to audit our data to make sure that it is actually correct?”
We needed both people and processes to ensure that data was validated. We built in controls for auditing the data, alongside reviewing the technical integration to ensure that the process of capturing and uploading the data was privacy-safe, efficient, and accurate.
This meant a shift in our organisational approach. We needed to ensure we were translating data into insights, and insights into action. This required bringing teams together and removing silos. To ensure data is ultimately creating value you need to connect it to a very clear business direction. All teams need to understand the action that you want to take, to ensure the right data and insights are provided.
Once we were assured of our data quality, we started to tackle new use cases.
A supernova data analytics use case
The infrastructure we have built enables us to quickly and easily implement advanced use cases supported by AI. One such use case was around customer sentiment.
What was surprising to me is that we took much longer to discuss and solve challenges around customer privacy and anonymity than we did on the actual implementation of the AI driven algorithm. The actual implementation of the algorithm to create a sentiment score was completed in just two weeks because of the robust data already available to us.
The project saw us look into global customer service tickets, translate them into English for ease of comparison, and then run a sentiment analysis to be able to group them into topics (e.g. returns, delivery, etc.). Part of this process was also to attach a sentiment score to each review. With that we can see how happy customers are with delivery, for instance. It also allowed us to take action on problem areas quickly. For example, if there is a payment method that is not working, we can see it directly reflected in the sentiment analysis and act immediately.
Star-studded new data sources with a loyalty programme and app
Being able to combine data from different digital sources was one thing. Being able to marry online interactions with offline purchases, or indeed offline interactions and online shopping, was another.
One of the ways that we wanted to tackle this data gap was through a customer loyalty programme.
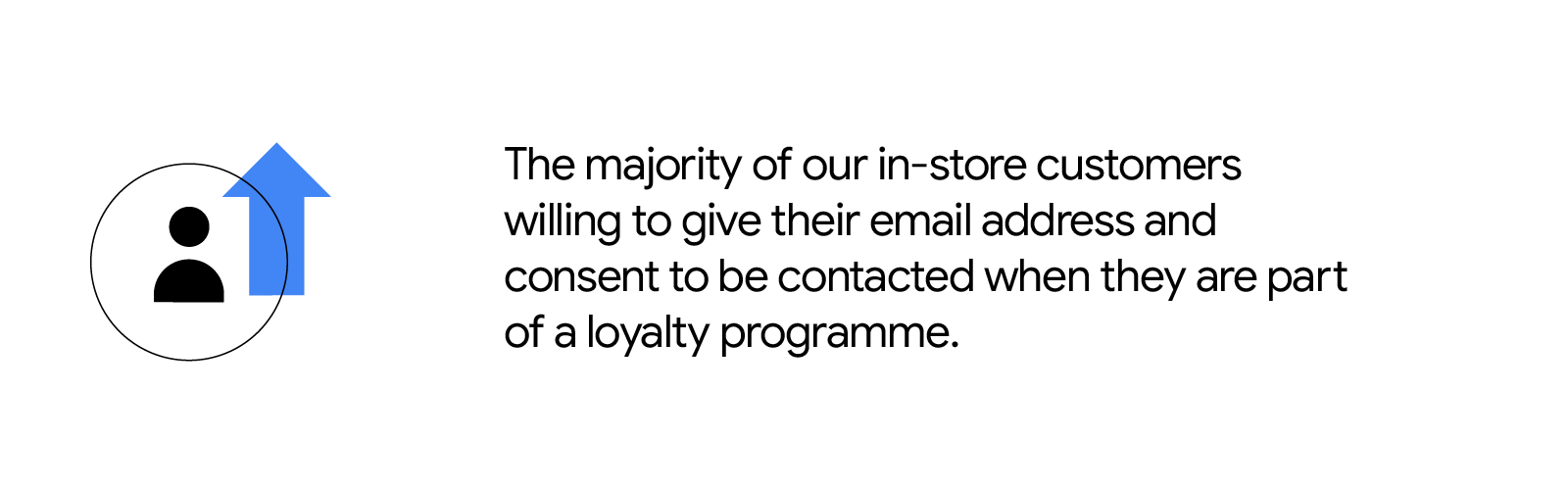
We found that we are able to get the majority of our in-store customers willing to give their email address and consent to be contacted when they are part of a loyalty programme. This information helped us to correlate their offline transactions and link them to online transactions via an email address.
We’ve also invested in our app to enhance customer experience and loyalty. We know people often browse on an app and then visit a store, and vice versa. Shoppers still value the personal experience they get in stores. We use our app to reward our customers by giving them early access to sales.
Travelling at lightspeed towards predicting customer lifetime value
So, what’s the next step on our customer data journey?
One thing we’d like to look at is a move towards a higher degree of segmentation and personalisation across the whole customer journey. Making sure we get the right email with the right content to the right customer at the right time.
As I said at the start, at G-Star we really see the opportunities in first party data. We may never have our full customer data and you have to accept that and find a balance between 100% complete data and enough data to create insight and action.
But there are lots of things we can implement to navigate these challenges, like incentivising customers to consent to share information with us and ensuring messaging to the customer is spot-on. By using consent well we can build trust with our customers, which hopefully leads to brand loyalty.
My advice to anyone else looking at how they can bring their data together would be: start with ensuring all your teams understand the wider business goals. From there we always ask: what is the data showing and what would the expected impact of this decision be? While data will never give you a definitive answer, it does provide strong direction, which means that we can always take data driven insights into account when making decisions.
Whatever we do next will be very consumer focused and driven by our data. Always asking and being led by the question: “What is our data actually telling us?”