Dr Ramla Jarrar is chief executive officer at MASS Analytics, a software company specialising in marketing mix modelling. They help businesses understand how to attribute sales to the right marketing investment.
As the cookieless era approaches, the measurement industry is looking for ways to plug the data gaps – and they’re increasingly turning to marketing mix modelling (MMM).
MMM enables businesses to measure and improve the impact of media and marketing efforts, all while respecting privacy regulations. This makes it one of the most attractive measurement solutions currently on tap, with many brands even bringing it in-house.
But there are misconceptions about the prerequisites a business needs to get started on their first MMM project, and indeed what type of data produces the most actionable insights. And for the creatives amongst us, there’s also the concern that it’s all just too much science.
To help you better understand the opportunities that come with the approach, we’ve broken down three of the most common myths about MMMs.
3 myths about MMM
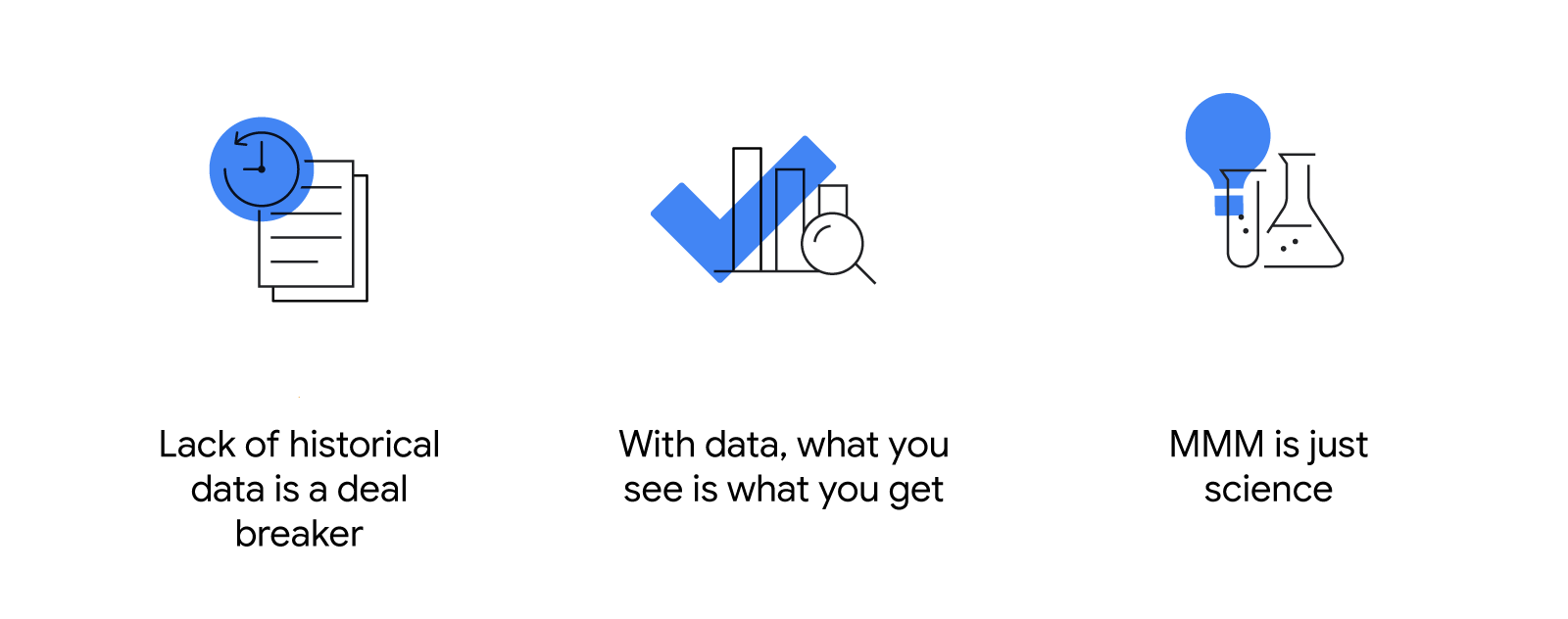
Myth 1: Lack of historical data is a deal breaker
It is common perception that MMMs can only work for companies with a long track record of historical data. This might have been true a few years ago, but contemporary techniques offer solid results even when a dataset does not cover the traditional requirement of three years worth of data.
That’s not to say that a lack of data history isn’t an obstacle; in order to detect interactions between drivers and outcomes, an MMM needs to have enough data points for each. But modellers can trick MMM into thinking that more data is available by using more granular data. For example, instead of looking at the relationship between all video impressions and sales that happened in the entire country, modellers can use data broken out by region, customer segment, or product line and then stack these datasets on top of each other, thus multiplying the overall number of data points.
To put it simply, if one year of data is available on weekly collection, a model will have 52 data points per variable. But if the same data is broken out by three customer segments, 156 data points become available – this is a big improvement for the MMM study.
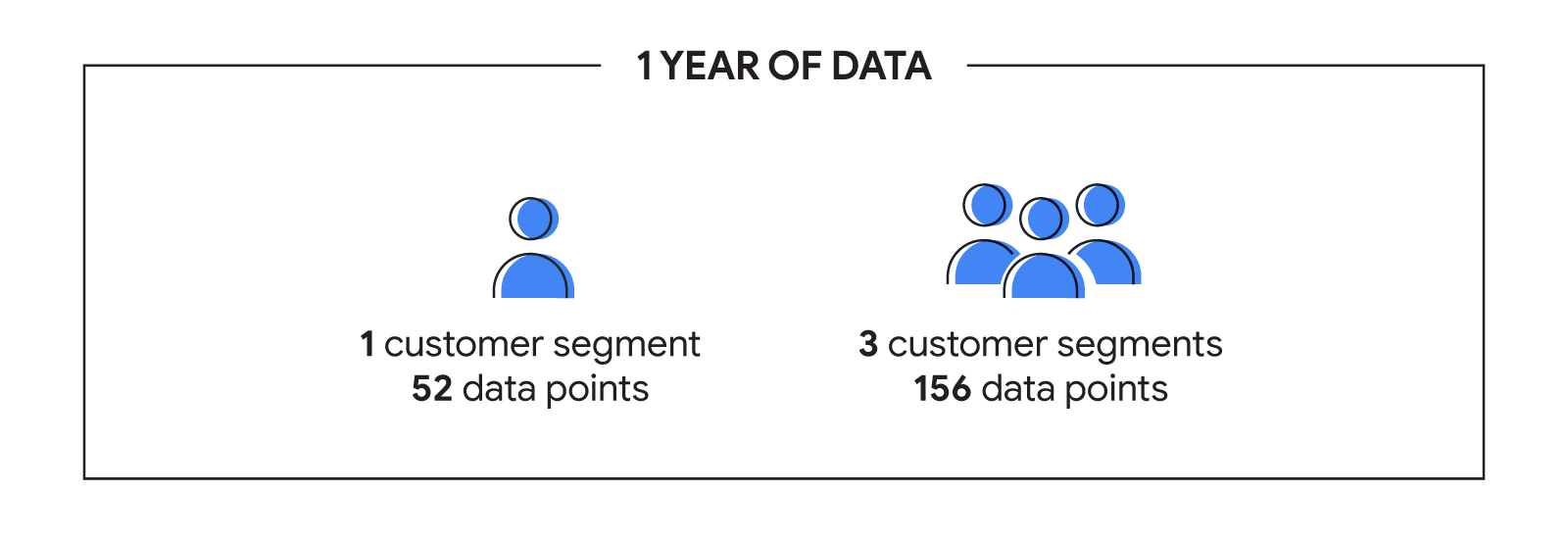
This approach can change the game completely as it means that a lack of historical data no longer has to restrict a company from running an MMM.
Myth 2: With data, what you see is what you get
In most cases, raw, unprocessed data is not usable. And ingesting it in a model, no matter how elaborate and mathematically sophisticated, will not lead to actionable results. Every variable has a story behind it that cannot be told through the raw format of data.
To fully understand what each variable represents, analysts must interpret the data to make sense of consumer behaviour, and address the business questions at hand. This will resolve one of the most challenging steps in the quest of acquiring meaningful insights.
For example, US-based chocolate manufacturer, Hershey's, recognised that different types of YouTube ads had different roles to play in the customer journey, so they measured ROIs by format type.
Every variable has a story behind it that cannot be told through the raw format of data.
This level of granularity has helped the Hershey's team establish that a mix of YouTube’s TrueView, Google Preferred, and six-second bumper ads was most effective in driving sales for Reese’s Peanut Butter Cups. As measured by marketing mix modelling, the retail ROI for The Hershey Company's portfolio of brands increased 40% year over year from 2017 to 2018. This shows the power of using an MMM to translate raw data into actionable insights, with impactful results.
Myth 3: MMM is just science
Of course MMM is rooted in science, but there’s an art to it, too. Your team or the tools you work with need to understand how marketing and media work. Otherwise, just like with art, it would be like asking a brilliant painter to produce an accurate portrait of someone they have never met.
No AI algorithm, no matter how advanced, can account for missing context in data. People are key to MMM success.
MMM requires a deep understanding of the complex modern-day consumer behaviour and media landscape. With the latter in particular, analysts need to be able to choose the right variables to add to the model depending on the vertical, the company, the market, and the consumers targeted by the modelled product. They need to understand how responses to a given media change based on elements such as geography or even a sub-segment within the targeted audience.
Other media-related factors that analysts should have a good grasp on are campaign elements such as message, format, and objective, as well as the media strategies of competitors. No AI algorithm, no matter how advanced, can account for missing context in data. People are key to MMM success.
When auditing the models of one of our telco clients, MASS Analytics noticed a massive gap in the offline sales model. When we dug further, we found out that they hadn’t included sales commissions. Only once we added this variable into the mix the model was able to recognise the true effect of impact on sales.
So saying that MMM is data science is not a complete myth; it is just half the story. And even though MMM has adapted to the latest changes in the measurement industry, it’s clear that its evolution is still clouded by some outdated myths. But taking the time to better understand these will take away barriers, unlock potential, and ensure a future-proof technique for businesses in all industries.
3 quick tips to get started on MMM:
- Defining the scope of the business questions is key. The initial build can allow to answer general business questions but as the in-house team gains in maturity the richness of the data collected and the results granularity will improve over time.
- Check data history and granularity. How far back have you been recording business outcomes like sales, brand KPIs, leads, registrations, contracts, that are relevant to your business? Can you multiply your data? Is data available by segment: geographies, customer groups, brand lines?
- Take stock of skills and knowledge in-house. Do you have skilled data scientists? Do they know enough about how marketing and media work? Do they have previous experience with MMM? If the answer to any of these questions is a no, consider partnering with a third party to either commission a full MMM, licence a tool or provide consultation.