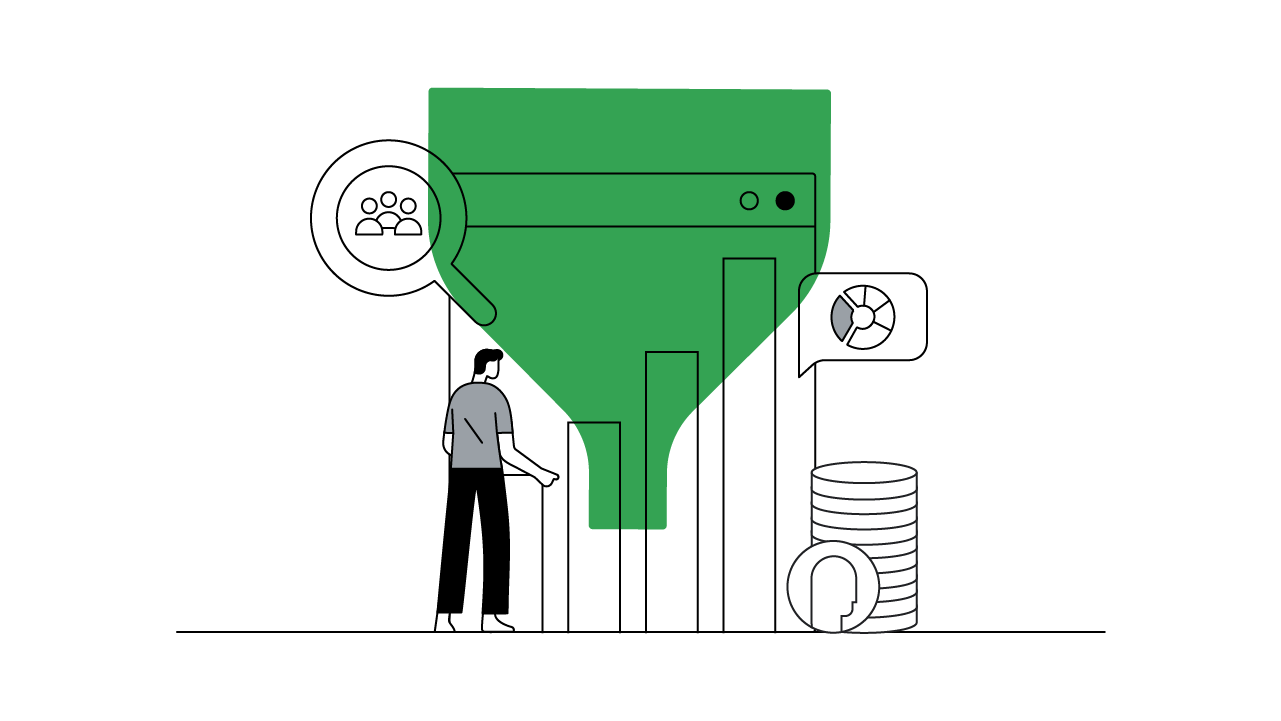
As advertising technology becomes more sophisticated, knowing how to pull useful insights from incredibly large amounts of data is becoming an important competitive advantage. Many brands still use lead volume as a way to optimize campaign spend. But as customer behaviors change and marketing budgets shrink during the pandemic, it’s crucial to leverage data and identify marketing metrics that will help drive the most profit.
To go beyond digital marketing ROI and increase profitability, brands must understand the small actions people take along the customer journey. Knowing what causes people to purchase or lose interest can help brands make smarter investment choices that lead directly to profit. And thankfully, there are solutions that can help.
The latest tools for automation and machine learning in digital marketing enable you to turn vast data signals into meaningful real-time action at scale. Here’s how three brands used these tools to make sense of their data and optimize their ads for profitability.
How to use customer signals to find the most profitable prospects
Start by using tools that analyze data signals to help you understand your most profitable customers. That’s how ZEE5, a video streaming service in India, brought more subscribers to its platform.
ZEE5 moved its ad campaigns to Google Analytics for Firebase (GA4F), which provides unlimited event logging and collects the data in a single place. This enabled it to analyze various data points from existing subscribers, such as location, device, and browsing behavior, and build a model that identified its two most valuable subscriber segments: reinstalling subscribers and users acquired from watching a show’s trailer.
Because GA4F automatically connects with Google Ads, ZEE5’s campaigns started bidding higher on prospects who share the characteristics of people more likely to subscribe and with higher lifetime values. It also began crediting campaigns for any reinstalls after 30 days of uninstalling instead of the previous six months to optimize its campaigns for the high-value segment of people reinstalling.
Its model also continued to be refined by machine learning, using performance data that was fed back into Firebase, which empowered ZEE5 to further optimize Google Ads. By focusing on the most profitable audiences, it saw 125% higher transaction value with 2X more total subscriptions.
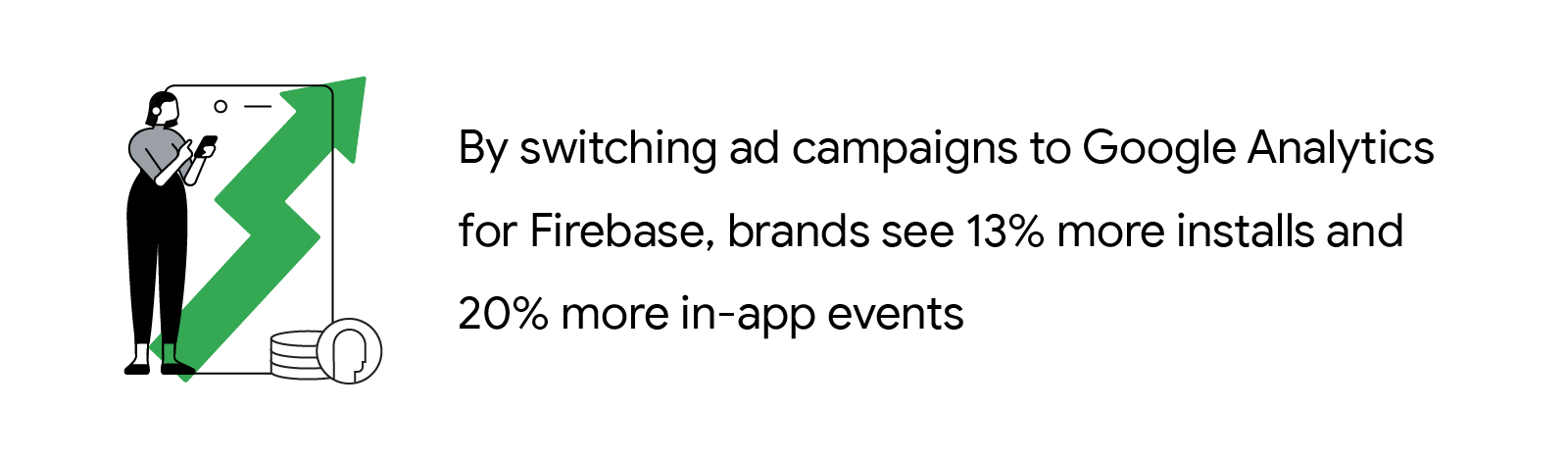
Brands using GA4F have more signals about their users, so they can find and bid higher on the best prospects. As internal Google data shows, campaigns that switch to GA4F bring in more active users, with a 13% increase in installs and a 20% increase in in-app events, at no significant change to cost per conversion.
How to identify and prioritize customers who are likely to churn
Another profit-driven marketing approach is to use data to identify and nurture customers who are at high risk of leaving. When Voot, an Indian video-on-demand service, wanted to reduce churn among its paying audiences, it partnered with Google to get a better idea of which viewers were likely to cancel their subscription.
The team used Ads Data Hub to easily consolidate data across various platforms, including first-party Google Ads campaign data and third-party sources such as Mixpanel. Voot then used BigQuery to analyze the unified data, which comprised more than 10 billion data points across the customer funnel, and build prediction models of audiences likely to cancel their subscription. It examined everything from content watched to viewership behavior and traffic sources and used machine learning to identify segments and key attributes to watch for, such as new users who have only watched one genre in their first month of subscribing.
With data driving its user churn prediction model, Voot’s remarketing budgets are now spent on those who need an extra nudge to stay.
It then segmented the likely-to-churn audiences into smaller groups based on similar behaviors. Looking at patterns of content that people in related groups had watched, it chose specific shows to recommend to each subscriber likely to cancel the service. In an experiment, the group that received personal recommendations had 35% fewer cancellations than the control group.
With its custom churn prediction model, Voot’s marketing efforts are now informed by data, and its remarketing budgets are only spent on users who need an extra nudge to stay, not on those likely to return on their own.
How to optimize Search campaign bidding on higher-value customers
Analyzing data across many customer journeys can help you identify valuable consumers who are worth your campaign investment, even before they become a lead. Oyo, an online hotel booking site, had always measured campaign performance with cost-per-lead. But when the pandemic put additional pressure on the hospitality industry, it realized many leads weren’t converting, so it decided to focus on high-intent audiences likely to complete their purchases.
To maximize sales instead of leads, Oyo shifted its Search campaigns toward value-based bidding, which enables higher bids on customers who are likely to bring more profitable outcomes. It also continued to feed first-party conversion data such as hotel stays and frequency along with transaction values back into the machine learning-powered campaigns.
By taking advantage of machine learning in marketing tools to make sense of the various data signals, Oyo was able to learn which of its customers were most valuable. In turn, it invested more of its budget toward reaching high-potential customers and improved its return on ad spend on Search campaigns by 75%.
Brands are missing out on profit by not connecting advertising spend to data across the entire customer journey. With the latest marketing tools, such as GA4F and Ads Data Hub, however, you can easily consolidate and analyze various consumer data signals with machine learning and put your marketing budget to work in smarter ways.